Attribution & Measurement Landscape 2022
Prohaska consulting published the first attribution and measurement landscape overview:
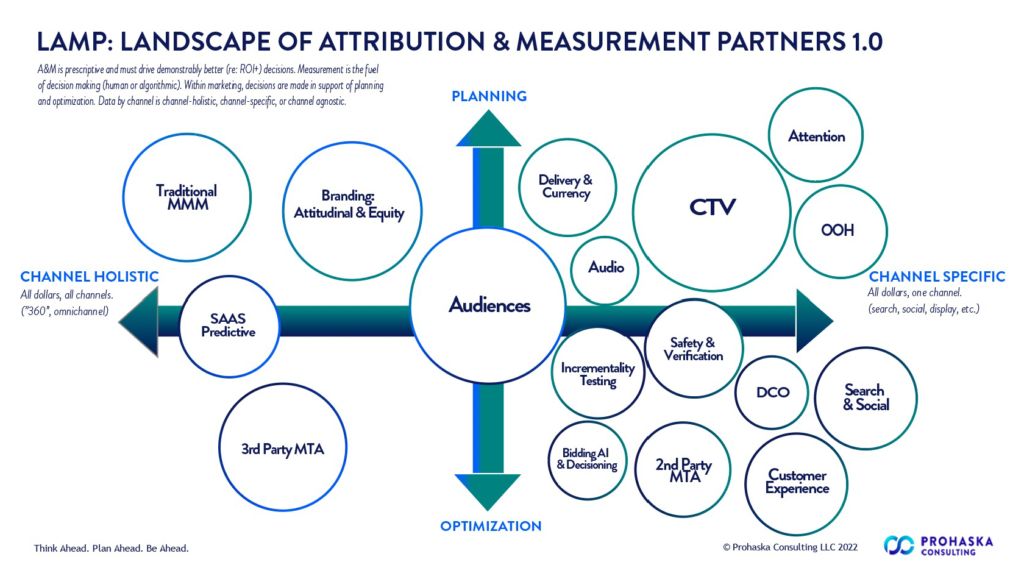
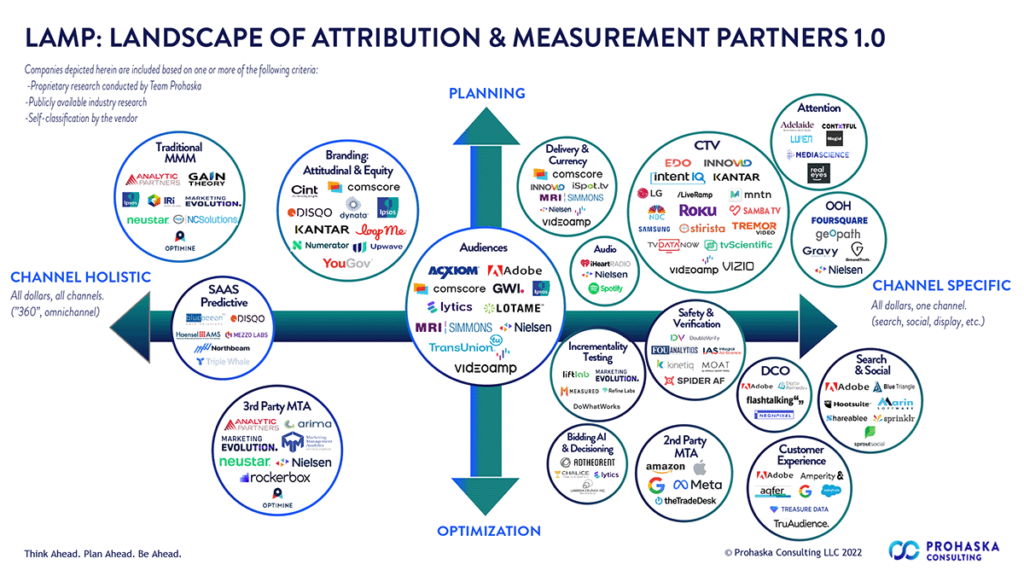
The mobile app market is in a frenzy and oversaturated. It is becoming harder to retain the attention of users these days, and it seems like everyone’s ready to hit the “uninstall” button at any slight irritation. Marketers seek to develop the best user acquisition strategy to be among that 0.5% of apps that succeed. Yes, the success rate of mobile apps is this low; according to a recent study, 99.5 % of mobile apps fail to become financially successful.
Interestingly, still more apps are launched every day to add to the existing number. Recent findings show that, in the first quarter of 2021, over 5.7 million different mobile apps were uploaded to all top mobile app stores combined – and more are added daily.
In another report, in 2021, approximately 230 billion mobile apps were deployed across various mobile ecosystems. This demonstrates that the mobile app market is, indeed, expanding at an exponential rate.
With these reports, there is no doubt that mobile app marketers face fierce competition due to impetuous market growth.
Today, it is no longer enough to develop or launch a mobile app. It is imperative, early on, to incorporate effective user attraction strategies into a marketing plan.
To succeed in this space, as a mobile app marketer, you must be meticulous with your user acquisition strategy. You’d have to utilize all possible approaches to attract a good percentage of users to your app(s).
In this article, I’ll explain how user acquisition works in mobile and will provide you with strategies for promoting your apps.
Factors that Influence User Acquisition in Mobile
The term “mobile user acquisition” refers to the number of new mobile users who can be converted to achieve an app’s goal. This goal could be to install, purchase items through the app, provide personal information, sign up for news, etc.
There are several tactics that you can use to achieve these goals; however, in order to be effective, the tactics that you choose must be tailored for the mobile environment. If resources are available, a more diverse acquisition approach can also be implemented to achieve really spectacular outcomes.
According to reports, 80% of users abandon an app after about three months. Over time, this has become a common occurrence and it is now considered normal. As a result, if you want to retain new users and keep them engaged, you must consider the factors that drive user acquisition on mobile, as outlined below.
Ability to promote and sustain a satisfying user experience
Your app must be fully optimized; by this, I mean the ability of your app to be lightweight (for quick download). The user experience must be impeccable as well – the app should load quickly, use little battery power and not consume too much internet bandwidth.
Also, paying attention to your target audience’s complaints and recommendations in the app store and fixing the technical glitches on your app can go a long way in enhancing your app’s user experience and keeping your app installed on users’ devices. An example is Uber and UberPool – both leverage users’ ratings to optimize their services.
Ability to offer value and address users’ concerns
What is the goal behind app development? To increase the number of app installations and generate income; however, you will only be able to make money from your app if you can deliver genuine value to your customers. Therefore, while planning out the app, you should think this and clearly state how users will benefit from your app. How does it address a particular need? When a need is met, users will keep your app installed for a long time.
Capacity to compete ethically
Your app must have a unique value proposition that gives it a competitive edge over other apps. Where this is lacking, you risk losing your app users to the competition. Is your app more efficient than others? Is it possible for your app to deliver a service that no other one can match? Can users get a solution quicker with your app compared to the alternative ones? Would users need a discount if they use your app? You should have answers to these questions before the app is developed.
When developing a customer attraction strategy for mobile apps, list the essential features you offer to your customers and emphasize one of them. If the benefit isn’t immediately apparent, the app won’t be able to compete and earn money. Advertising can be used to develop a value proposition that is appealing to customers. A 10% discount on every app order is an obvious and understandable benefit to a user. Benefits should be obvious to the users – only in this way will you be able to increase user retention.
Organic mobile marketing user capture
Organic mobile marketing user acquisition is about using organic traffic to acquire customers – in this context, mobile app users. This technique has been found to work best for business to consumer (B2C) businesses that have sound knowledge of their target audience. Popular organic mobile marketing user acquisition sources and/or channels include websites, blogs and social media sites. You can adapt the strategies mentioned below to get the most out of your organic mobile acquisition efforts.
Properly optimize your mobile website
For the best results, it is important to enhance your landing pages and mobile website for specific SEO keywords. To maximize your ad spend, a landing page should be built at the start of a mobile ad campaign to accept visitors and ensure that details like prices, coupons and dates on the creative match those on the landing/website.
However, all efforts should not be spent on SEO, as optimizing your blog for search, volume and placement of keywords should not be at the expense of the quality and usefulness of content. To get started, content must be accurately displayed on mobile devices, followed by creating a content marketing plan for the blog.
Select the right ad formats that complement the content
Publishers whose mobile websites have high traffic don’t want to miss out on the possibility of earning more revenue from their content. This is because for each ad view amassed, they receive a share of the advertiser’s budget. These programmatic advertising systems make the process of ad placement easy by linking global mobile publishers with advertisers; this way, publishers automatically find the best option for mobile inventory monetization and advertisers instantly reach suitable publishers who support the ad formats that they serve.
It’s worth noting that mobile ads may appear within apps or on mobile web browsers. While mobile web campaigns may share similar ad formats with desktop web campaigns, the approach in choosing the best ad format may be different and depend on the objectives and screen resolutions.
When developing in-app or mobile web creative, it is essential to adhere to proven formats, applying the appropriate heights, weights and resolutions, as well as including CTA and links in the banners to direct the audience to the landings and boost user acquisition.
Factors that Influence User Acquisition in Mobile
Demind Side Platform (DSP) can help you to advertise apps across various mobile channels, as it is a viable tool for mobile app user acquisition.
One option is to allow the system to use RTB protocol that will automatically find desired audiences, bid on impressions and then serve the ads on mobile inventory. Another option is using programmatic direct deals, where you deal directly with the publishers without participating in the auctions. When done right, either method can give you the best return on your ad spend.
Employ targeting
Every audience segment that you try to reach may have a specific set of parameters that you can target on a DSP. DSP, with its number of targeting criteria, can help you to individually address every audience segment. Among the targeting options to consider are the browser, the geographic location, the connection type, the device brand GPS, the IAB categories, the language, the gender and the age.
Explore media ad formats
Using a mix of image/banner and video ad formats can greatly enhance your mobile app’s user acquisition efforts. Banner ads are arguably cheap, easy to use and supported by almost all DSPs, which makes them the preferred option for app monetization – especially for free apps. You may also use video ads that last up to 30 seconds to reach outstanding completion rates. Other ad formats that you might want to consider include native, rewarded video ads and playable interactive formats.
Make use of retargeting
You can do mobile retargeting via DSP, thereby re-introducing your offer to people who once visited your website but didn’t convert. Additionally, retargeting helps you to greatly reduce the chances of ad fraud, because you get to reach out again to users who visited your website or app so that you can be sure all impressions are viewable.
Influencer marketing as a mobile user acquisition approach
For the past few decades, social media influencers have paved the way for effective mobile marketing. These bloggers, celebrities and blog owners can use their popularity to promote various products and services.
This advertising strategy is not restricted to just a person but can involve an entire organization, stimulating more audience interest. The advantage of using influencers for marketing is that they can connect with people exceptionally well.
Below are some tips to get the most returns from your mobile user attraction strategy with influencer marketing.
Define the target audience. Targeting a particular audience for advertisements is of prime importance to the effectiveness of the mobile app acquisition strategy. If you involve an influencer, it should be one with an audience you can advertise to. For example, a popular and professional athlete would make a good influencer for a sports product, like a shoe.
Choose the platform. When choosing an influencer, you should also consider the platform(s) where your product will be advertised. YouTube, Twitter and Instagram are great choices, but their audiences differ. Knowing the platform where your target audience congregates can help you determine the effectiveness of your promotion.
Knowing how to measure effectiveness. Before and after engaging an influencer to promote your ads, you have to consider different parameters that you can use to determine whether or not the promotion is/was successful. Comparing the number of people who saw your ads to the number of installs or sales is a good way to determine the effectiveness of influencer marketing.
Finding new users through email marketing
Email marketing is of great relevance for any successful mobile app user acquisition. The effectiveness of email marketing, by far, outweighs that of social media advertising because of the high rate that people click email links, especially on mobile devices. Because of this, you should format your email newsletters to appear perfectly on all mobile devices.
Be concise. No matter how interesting your offer is, nobody likes to read an email newsletter that is too long. So, while utilizing email for your marketing, keep the message short and garnished with perfectly optimized pictures. This method has helped Uber increase its app user acquisition by 443% in just three years using email marketing.
Personalization. Different audiences find different things appealing. Since apps are usually advertised worldwide, you must ensure that you take this into account when sending out your email newsletters. Divide your email newsletters into different segments based on user interests, preferences and geolocations. Create several versions of email newsletters for each segment. This will help you send only targeted emails to specific users at the right time.
Include deep links. Some users need a prompt or a link directing them to the correct app. This is where including deep links in your email newsletter will be helpful. Deep links in your email newsletter will increase downloads and allow you to track the effectiveness of your email retargeting campaigns.
Conclusion
Understanding mobile user acquisition will aid you in developing a good marketing plan. I am sure that if you implement the recommendations from this guide, you will quickly attract new users to your mobile app and grow it to be very profitable. In addition, partnering with a demand-side platform would further enable you to optimize your user acquisition strategy. DSPs leverage programmatic advertising technology that allows you to channel your ads across various mobile applications and with optimal reach.
About ITP and Server-side tracking – Do you know how ITP (intelligent tracking prevention) is exactly affecting your tracking data and hence your customer journey analytics? We’ll go over how event tracking actually works: Client-side and server-side tracking, and also how they are impacted by ITP. Which are the benefits of each of the tracking options? Find it out in this video.
Chapters:
0:00 – Intro
0:10 – What is client-side tracking?
1:20 – What makes client-side tracking unreliable?
1:55 – What is ITP exactly doing?
2:40 – What does this mean for our data analysis?
3:55 – How can server-side tracking help us?
You can also take a look at the IHC attribution introduction video and the results analysis and basic insights
Last week, just before its huge earnings miss was attributed to Apple’s privacy restrictions, Meta published this article encouraging advertisers to run incrementality experiments to get an accurate read on Facebook campaigns in the post-iOS 14.5 world. If it sounds familiar, well that’s because the narrative is nearly identical to the story we’ve been telling for years.
So, why are platforms just now coming around to acknowledge that incrementality testing is the most accurate method for measurement?
Apple is a convenient distraction and scapegoat for platforms to blame their current measurement and tracking issues on, but the truth is that Facebook reporting was never reliable in the first place. Pre-iOS14.5, the flawed last-touch attribution methods long used by most ad platforms made them look good. Now, increasing data restrictions are simply exposing platform attribution for the broken system that it is.
Before the collapse of user-level tracking, incrementality measurement often revealed that platforms like Facebook were overreporting conversions. The propensity for ad platforms to claim more credit than they deserve was due to the known inherent flaws of using last-touch metrics. By counting every conversion an ad was in the path, Facebook ignored the fact that some of those events would have happened anyway, not to mention the impact of ads from other vendors that they have always been blind to. The amount of extra credit could vary widely by audience type – think prospecting (mild) vs. retargeting (heavy).
Today, having lost the ability to track conversions for a significant segment of their users, Facebook is now vastly underreporting conversions, and testing for incrementality is suddenly the ideal approach to measurement. Incrementality experiments and geo-matched market testing can prove the value of campaigns on the platform while their own reporting systems cannot.
In an industry dizzy with inconsistency, incrementality is the one thing that remains consistent. Underreporting? Overreporting? Oversaturated? Underfunded? Testing for incrementality will consistently deliver valuable and actionable insights.
We work with more than 100 DTC brands, and every single one of them has some level of media budget being invested into Facebook. Some advertisers we work with are using incrementality testing to justify their continued investment in Facebook or to expand into things like reach optimized campaigns, knowing their campaigns are performing better than Facebook attribution would lead them to believe.
Other brands want to diversify away from steadily rising Facebook ad costs and are using incrementality experiments to test and scale into new channels like TikTok, Snap, Pinterest, or CTV. With incrementality and geo testing, marketers can spend a small fraction of budget to understand how allocating new significant investment into these channels will pay off.
We’re glad to see the platforms come around on incrementality and geo. Not just because they are helping us spread the word, but because it really is crucial for DTC brands – and for an industry that has been plagued with trust issues as far back as I can remember.
Incrementality measurement doesn’t require tracking at the user level, it can be run independent of potential platform bias, it’s rooted in proven scientific methodology, and it can provide ongoing insight at a tactical level so advertisers can continuously move dollars to where they drive the greatest business impact.
I’m not saying decisions by Apple, Google, and others haven’t had or won’t have a significant impact on the media industry and businesses that operate within it, but when it comes to platform attribution, nothing has changed. Facebook and other walled gardens were perfectly happy with a broken last-touch system of measurement when it provided results in their favor. My, how the tables have turned.
In any event, it has never been more clear that incrementality testing and experimentation could finally put to bed a decades-long struggle, for platforms and marketers alike, and become the standard advertising measurement approach that is useful and acceptable for everyone involved.
Measured.com – You can read the full article also here.
Attention will become the dominant advertising currency
There are two key reasons 2022 will be the year attention becomes the dominant advertising currency.
Firstly, disruption, from tighter privacy controls to cookie deprecation, is impacting how brands target consumers and measure performance in their digital campaigns. More than ever, brands in 2022 will be looking for other measures they can take to gauge performance in an accurate, privacy-friendly way. Rather than buying inventory blind, adding attention to the media measurement mix can help ensure accountability over spend.
Secondly, brands will continue to want to drive outcomes from spend. Putting it another way, if you’re advertising a new product, the goal isn’t to drive a specific number of impressions, ultimately, it’s to drive an outcome, like a sale. Attention metrics – such as audibility and screen touches, provide powerful new sets of data that are more predictive of an outcome – which brands can use to optimise their campaigns. Likewise, brands can achieve this without tracking consumers or requiring personal information.
The shift in focus to attention metrics, alongside advancements in areas such as contextual targeting, is the natural next step in providing advertisers with better quality inventory and stronger results. Privacy regulations and the deprecation of cookies are undoubtedly accelerating this change. However, the shift to attention as the industry’s new currency isn’t only reactive, but grows out of verification solutions. Advances in verification has continually improved the baseline quality for ads by tackling fraudsters, boosting safety, and providing brands with clarity over their ad performance.
Exchangewire.com – You can read the full article also here.
Marketing communications in the digital age evolve by the day. Getting and retaining customers is all about providing a great customer experience from first interaction throughout the buyer’s journey. Today, consumers wield all the power, and with just a quick skip, swipe, or scroll, they determine whether a brand stays relevant or not.
As marketers explore strategies to drive the right kind of engagement online, one critical discussion that always comes up is whether they should opt for multichannel or omnichannel marketing. But, there’s one thing everyone can agree on: consumers expect a quick and seamless experience across all digital marketing touchpoints.
That said, let’s discuss multichannel vs. omnichannel marketing to determine which of the two can provide a better customer experience. After all, great customer experience yields a competitive advantage.
Omnichannel vs. Multichannel – What is the Difference?
«Multi» suggests «many» while «omni» suggests «all». However, the fundamental difference between multichannel and omnichannel marketing has little to do with whether you’re engaging customers in several touchpoints or all touchpoints as they move down the sales funnel. Rather, it has to do with integration.
In multichannel marketing, the goal is to guide a consumer towards a particular goal. It focuses on getting them from one stage of the buyer’s journey to another by sending a standard message through individual channels. Let’s say you’re issuing a promo discount code for new users; multichannel marketing involves you advertising the same promo code on several channels like your blog, social media pages, and email newsletter.
Multichannel marketing segments direct and indirect customer interactions. Direct communications are where the company proactively reaches out to the customer through direct mail or message. Indirect interactions include content pushed through blogs, websites, or social media.
Even though the marketing channels belong to the same entity, they’re inherently isolated from one another. Each channel works in a silo, with its own strategy and goals. As a result, brands that rely on multichannel marketing tend to run into miscommunication and style inconsistencies between channels.
For customers, multichannel marketing can create a confusing, impersonal experience that leaves them feeling frustrated.
Now, unlike multichannel marketing, omnichannel marketing has the customer at the center. It involves the seamless integration of branding and messaging across all touchpoints to create a more impactful customer experience. An omnichannel communication platform allows customers to determine how they’ll interact with your brand by offering:
In other words, marketers take a step back and let users define the journey for themselves – but the trip is tailored to their likes and preferences. For example, a user might get an email announcing a new product that goes perfectly with another they’d previously bought. Instead of relaying the same message through other channels, your strategy might involve offering them a promo code for that exact product on Twitter and having them see a video of someone using the product on Facebook.
In both cases, the goal is to get customers to take a desired action (using a promo code). The difference is in the journey options you provide.
Multichannel marketing
-Reach user through a maximum number of channels
-Brand is on every channel, but each channel may have different messaging
-Doesn’t use the advantages of using multiple channels in tandem
-Fragmented, inconsistent experience
Omnichannel marketing
-Gives users a seamless experience across every channel
-No matter which channel is used, the message is consistent
-Must be used with analytics to individualize experience to each user’s needs
-Seamless, consistent experience
So, Which Is The Better Option?
Brands that choose multichannel marketing do so for one reason; it’s pretty straightforward. All you need is one main message and a call to action. However, this can be alienating to customers as it doesn’t take their needs, preferences, or stage in the sales funnel into consideration.
An omnichannel customer experience could be the brand differentiator you need. An omnichannel marketing platform nurtures leads and user engagement by offering audiences a diverse set of messages, incentives, or buying options, bringing the following benefits:
·A better user experience – Omnichannel communication focuses on the individual customer experience instead of the channel. It allows for a cohesive customer experience no matter how or where a customer reaches out.
·Increased revenue – Focusing on omnichannel customer engagement ensures a seamless experience for shoppers, making them more likely to buy from you.
·Creates a consistent brand image – Integration means you can create a cohesive brand strategy and identity across all channels, which leads to increased loyalty as customers will trust your brand more.
·Better attribution data – With omnichannel marketing automation, you’ll be able to identify which channels work best for your brand and when customers are more likely to engage with you on their journey. Such data can be used to improve brand strategy and build more targeted campaigns.
Support Customer Engagement Across Every Channel That Matters
Customer experience is an asset to your brand – make it work for you. As mentioned, many brands opt for multichannel marketing because it’s easier to execute. But, if you’re looking to captivate and engage your customers on all channels, you have to leverage insights and create messages tailored to their interests and where they are on their journey.
Omnichannel marketing automation platforms allow you to do this and more. You can create more targeted, effective campaigns and put your customers’ needs front and center. Use data insights to drive campaigns and aid in journey planning, all while executing effective omnichannel communications from one place.
Altcraft.com – You can read the full article also here.
Without a display campaign, your marketing strategy is missing a crucial feature: its foundation
As a leader in programmatic advertising, Genius Monkey knows that a well-rounded strategy includes display first and then other programmatic services added on to complement display. In fact, data shows that display campaigns are the backbone of almost every successful digital marketing media plan.
Study results:
Audio touches 9% of all conversions
Display touches 58% of all conversions
Video touches 33% of all conversions
OTT/CTV touches 22% of all conversions
Retargeting, whether that be display, video, or a mix, touches 27% of all conversions
The main takeaway? It’s essential to have a good mix of programmatic mediums, but display ads should be the foundation to every digital strategy. Why is this? Display has a larger reach and a clearer influence, which is clearly shown in that it touches 58% of all conversions.
Part of the reason display works so well is because display has a much less expensive cost-per-thousand impression than other programmatic mediums. This means you pay less for every time the ad is displayed, allowing for much more impressions, branding, and the like. Here are the costs Genius Monkey observes for such mediums:
Display: $2 – $4 CPM
Video: $10 – $22 CPM
OTT: $29 – $55 CPM
Audio: $21 – $37 CPM
Part of the reason for the lower cost is due to available placements, which also explains the better performance-because more placements are available, display ads will have better reach. This means that for every video placement a website may have, there are about three more display ad placements available on that site.
Here’s what we have found: if a marketer only uses online videos, they are limited on the amount of websites, apps, or inventory they can place those videos on. Online video ads also cost at least 8x more per impression than display ads, allowing less impressions, and in turn branding, extending the conversion timeline. This is why video, audio, and OTT should be used as a support to display advertising.
With all that said, we aren’t discouraging you from running other mediums, because those other mediums contribute to conversion. In fact, our data shows that when a user is running all 5 of our services at once, the average conversions that occur are touched by at least 3 different mediums. This means 56% of users who converted saw at least three different ad formats. In fact, different mediums can also help push people through the sales cycle (conversion funnel) faster, and have other effects that can be strategically utilized when added to display.
In other words, Genius Monkey has found that by adding in mediums in addition to display you can potentially shorten the conversion cycle, drive more conversions, drive different audiences, and drive other beneficial metrics. However, by replacing display and only running those other mediums, you lose the foundation to your marketing and will tend to see drop-offs in performance. What does this really mean? Other digital marketing mediums should be used to supplement display-not replace it.
See how Genius Monkey did just that by shortening a client’s path-to-conversion cycle by adding in programmatic OTT video advertising.ç
A Real Example with Advanced Training Institute
Through their agency, Magic Marketing, the Advanced Training Institute (ATI) began running ads with Genius Monkey for just over a year and a half, using display and video to fuel their ad efforts. One challenge they wished to overcome, however, was the length of their conversion cycles. Relatively long conversion cycles are normal for education marketing, but ATI wanted to see if improving their cycle length was possible.
After establishing a campaign baseline through the use of DISPLAY and online video ads, the team wanted to test OTT ads to see how it affected the conversion cycle. After adding OTT to the mix, attribution showed that users were converting faster than previous years. Here are the results:
Path to conversion became substantially shorter
Users who took over 90 days to convert decreased from 63.5% to 35%, a 45% improvement
Users who took less than 2 days to convert improved from 5.1% to 22.6%, a 343% improvement
These results show why you should use additional services to supplement display advertising. Diversity of ad spend and media buying is key to a successful digital campaign; however, it should all lead back to display ads, as display ads provide the foundation of success. Without display ads, the foundation that you are building on is far less stable and you will never reach the heights you otherwise could.
Digitaljournal.com – You can read the full article also here.
While data privacy concerns and the regulations designed to address them have been on the rise for a decade, the bold policy moves recently made by platforms like Apple, Google and Facebook have motivated marketers to seek better alternatives to a decomposing system of advertising measurement.
As with any industry problem this ubiquitous, solving the measurement dilemma for marketers could prove quite lucrative – and there is no shortage of vendors claiming to have the answer. As more brands embrace experimentation as a viable solution, many adtech providers have been quick to adapt their language to suggest they have just what marketers need.
At Measured, we are thrilled to see the industry warming up to incrementality testing and experiments. We began developing our methodologies and experiment designs more than five years ago, in anticipation of this very moment. However, marketers should take note: not all experiments are created equal – and very few of them measure the true incremental contribution of media to the business using your source of truth transaction data.
It’s vital that your experiments are rooted in proven scientific methodology and designed to provide reliable answers to the questions you need answered. Anything less could lead you down the wrong path, with serious business implications.
To ensure your ongoing media investment decisions are informed by reliable data insights, here are some critical features to consider as you evaluate potential partners for incrementality testing and experiment support.
· Are your experiments designed using proven test and control methodology?
Measuring the true incremental contribution of a channel, campaign or tactic requires experiments rooted in proven test and control methodology. Sending two campaigns to the same audience to see which one “sticks” is not a sound experiment
To be scientifically significant, deploying a clean, uncontaminated control cohort that is an exact replica to the exposed audience (test cohort) is critical – and not easy to achieve. Experiment design involves carefully selecting and controlling all the different variables, then collecting data in very specific ways to unpack reads for each cohort and apply them to a cross-channel reporting framework.
**If you are only making comparisons and not using a credible testing methodology, you aren’t really running experiments. To get meaningful actionable insight, you need experiments carefully designed to measure your desired outcomes, using your preferred metrics/taxonomy.
· Are you really measuring the incremental contribution of media?
If you’re only running tests provided by platforms, you’re only looking at relative lift by a publisher who is also selling you advertising inventory. More advanced levels of experiment design and testing that incorporate business transaction data from a source of truth like your ecommerce platform are required to reveal the true incremental contribution of media to a guiding metric like ROAS, CPA, LTV or sales.
**If you are only using self-reported last-click attribution data from the platforms and not reconciling it with source of truth business transaction data, you are getting misguided recommendations that are not based on true incremental contribution.
· Is geo-testing part of the experiment strategy?
While in-platform conversion lift testing is still available on some channels, the disintegration of user-level tracking has made them less stable and the reporting less reliable. Anchored on 1st party data, geo-matched-market testing is a near-universal approach for measuring incrementality on almost any platform. For channels that don’t provide control-cell capabilities, geo-testing is the only approach.
If a measurement vendor says they can experiment for incrementality on channels like Google Adwords, Facebook or Pinterest, but they are not using geo-matched market methodology, they are merely using an existing campaign as a baseline, not deploying a true control cohort. This can only tell you if one campaign is more incremental than another, not the overall incrementality of the channel or tactic.
Geo experiments don’t require user-level data, but they can still reveal the incremental contribution of media to any metric that can be collected at the geo level. Brands we work with that have run geo-experiments in tandem with Facebook testing have revealed that, while Facebook attribution has gone down due to shrinking attribution windows and ID resolution challenges, contribution has actually remained steady.
**If you aren’t using geo-matched market testing capabilities you aren’t getting a true read on many platforms. It’s the only future-proof method of measurement that will keep delivering results as ID tracking and in-platform attribution methods decline.
· Can you test for scale?
Along with understanding performance and the incrementality of various campaign elements, every marketer wants to know how much further they can take it. How much more can you invest in something before the law of diminishing returns sets in? Scale testing can identify saturation and opportunities for scale without risking budget.
**If you are not testing for scale, you are stuck with running full experiments, at full cost, until diminished returns are observed through wasted budget. Don’t throw money away.
· Are your experiments automated and your insights ongoing?
Many experiment providers expect the marketer to manually create and manage campaigns in the chosen platform, and then they simply tabulate the “results.” Not only is this process tedious and ripe for human error, it lacks the ability to execute these experiments at scale and over time.
Continuous testing at scale requires an always-on automated experimental design, campaign creation, and live result dashboards that can be tracked week to week, month to month, and year to year.
**If you are required to set up and manage campaigns and experiments on each channel yourself, you aren’t using experiments designed to reveal valuable actionable insights – you are just buying reports that package your data in a different way.
Experimentation is a must-have for growing DTC Brands
Using experiments to test the incremental contribution of media to your business is the best way to make informed decisions that fuel growth. But, beware of experiment impostors.
Only Measured delivers ongoing, reliable insights based on scientifically sound experiment designs that incorporate your source of truth transaction data. Everything is automated – ingestion and management of data from hundreds of sources, experiment design and implementation, and continuous reporting for confident, agile decision-making.
Thanks for reading – Want to learn more or schedule a demo? Get a Demo
Measured.com – You can read the full article also here.
In our new video we’ll talk about ITP and find out which is the best event tracking option for an optimal data collection. For us, building good customer journeys is essential for a smart attribution. Client-side or server-side tracking? Find our winner here, and let us know if you agree!
You can also take a look at the IHC attribution introduction video and the results analysis and basic insights.
It is usually the prerogative for the marketing team to drive inbound leads and get a larger percentage of the available online traffic to interact effectively with a brand. Inbound leads are leads that show interest in a brand’s products and services and they usually reach out with the goal of wanting more facts and information. If planned right, inbound leads or inbound callers can be made to convert faster than other traffic and audiences, this is where Call Analytics platforms can help.
Marketing Technology News: MarTech Interview with Michael O’Connell, VP Marketing at AnyRoad
Call Analytics systems can play a crucial role in closing the marketing gaps, especially lags between different channels. This can lead to better planning and handling of inbound leads while also enhancing the marketing-sales relationship and alignment within a company.
Marketers today can integrate call analytics data into their existing martech stack to get a more comprehensive view of their customer buying journey. Why have call analytics platforms become more important to marketing and sales teams?
A few of the top benefits of using Call Analytics Systems include:
Marketing Technology News: MarTech Interview with Christina Richards, CMO at Virtana
Enterprise call analytics platforms can be beneficial to both sales and marketing teams. Improved processes and implementation techniques can drive organizational goals and ROI and help streamline the movement of prospects.
Leading marketers and sales people have been using call analytics solutions to drive better engagement. Call analytics systems allows marketing teams to adopt a data-driven approach calling as a channel while giving them the right triggers to push conversions at a lower CPL.
Understanding How Call Analytics Platforms Work
Call analytics platforms work by routing incoming marketing calls to the right person or channel. These platforms assign unique tracking digits to each marketing sources making it easier to identify which lead is calling from which source. These tracking digits capture the caller profile and other attribution data like name, location and more.
Sophisticated AI powered call analytics platforms today analyse conversations and provide prompts based on it to marketing and sales people. These platforms often use speech-to-text technology to help teams breakdown call conversations.
The data that call analytics platforms today can generate can help marketers measure performance across channels and also assess the quality of their multichannel traffic to lead to better optimization, decisions and plans in the future.
Marketing Technology News: MarTech Interview with Johann Wrede, CMO at Xactly
Originally posted in Martech Series – You can read the full article also here.
You want to contribute? Please, we are waiting for you: